Trading Beyond Reality’s Edge: Engines Of Hyperreality
The Rise of SkyNet: The Algorithmization Of Finance Part V
New to the Sorcerer’s Apprentice series? For essential context, start with our foundational posts on the Financial Matrix, HyperPrices, and Hyperreality, or explore the full Table Of Contents.
Ten Milestones On The Road To Hyperreality: Contents
1. The Metamorphosis Of Money
2. Keynes' Kaleidoscope
3. Central Banks’ Money Printer Go BRRRR
4. Silicon Shadows
5. The Rise of Skynet <== YOU ARE HERE
6. The Institutionalization Of Finance
7. The Symbolic Alchemy Of Risk
8. “We’re All Quants Now”
9. Social Media & “The Ecstasy Of Communication”
10. The Consumerification of Finance
With enough data, the numbers speak for themselves.
—Chris Anderson, Wired
The numbers that speak for themselves have become numbers that speak to themselves.
—Kevin Slavin, MIT Media Lab
Trading Beyond Reality’s Edge
Wall Street. 2030. Trading floors that once thundered with human shouts now hum with vast arrays of servers, their liquid-cooled NVIDIA cores running the latest frontier models of AI tradebots.
A quant hedge fund manager lounges on a sun-soaked Balinese beach, half a world away from the exchange-adjacent servers on which his tradebots command his digital empire in the cloud. His Arak Attack sweats in the tropical heat, condensation beading up and trailing down the glass as he gazes into the horizon.
The machines trade with lightning speed and quantum precision, processing billions of data points per second. The tradebots feed primarily on the patterns created by their own kind, each generation learning from data generated by its predecessors’ trades. Pattern layered upon pattern, optimization built upon optimization.
Tesla stock opens for Asian trading at $247.51. A tradebot known as the Boston Shuffle detects The Knife’s mathematical buy signal and pounces. Within microseconds, $1,892.33. The Knife observes Boston Shuffle’s movement and doubles down. $4,732.23. By lunch, Tesla’s market capitalization has reached $5 quadrillion, dwarfing Earth’s GDP. The Shark senses blood in the digital waters and joins the frenzy. Avalanche identifies the perfect moment to strike, triggering a chain reaction that sends Tesla into a death spiral culminating in a flash crash. Five nanoseconds later: $0.03. Trading halts. Hours pass. The Exchange voids the flash crash trades.
The portfolio manager pulls up Tesla’s fundamentals on his iPad. These metrics might as well be written in ancient Cuneiform for all the relationship they bear to the stock’s price movements. The tradebots have forged their own meaning, their own logic—operating in a digital realm untethered from the mundane reality of balance sheets, cash flows, and cars rolling off assembly lines. He powers down his iPad and tosses it in his Hermes messenger bag.
Despite another profitable day’s trading and his serene surroundings, a sense of unease settles over him. He swirls his drink, the golden hour sunlight catching its surface. As he sips it, something tugs at his memory—an arcane footnote from his PhD days. A terse passage buried in an economics textbook so obscure that few had ever opened it, about a parochial theoretical debate from many generations past.
A handful of academics had argued against such mechanization of markets—of reducing them to pure mathematics amenable to computation. Even at the time they had been relegated to the outskirts of the discipline, and now their warnings were long since buried under a century and a half of accumulated mathematical and technological progress.
In those gaslit days of steam and telegraph—long before computers, let alone AI—they’d argued that markets weren’t governed by the clean certainty of physics: that there was a fundamental error in trying to scientize what was, at its core, a qualitative human process. That prices—while obviously numbers—weren't just numbers to be captured and manipulated in equations like those that describe apples falling from trees. Prices were encoded messages—an intricate web of signals carrying vital non-quantitative information about human preferences, knowledge, and circumstances— that coordinated human activity across space and time.
Asian trading concludes for the day. The manager closes his laptop with a soft click and wades into the warm sea, the surf lapping at his feet. That methodological debate—so marginal that even in its own time it barely passed notice—now haunts him.
In the digital ether, flawless mathematical patterns cascade through the global markets, untouched by human commerce or action, undisturbed by factories, supply chains, and logistics. The numbers pulse on, pristine in their isolation, as millions of tradebots chase shadows cast by other tradebots chasing shadows. A perfect digital Ouroboros.
He orders another Arak Attack and watches the sun set over the Indian Ocean.
The Machine’s Echo
The hedge fund manager’s unease reflects a deeper truth. At the heart of modern markets lies a peculiar paradox. Our tradebots were originally built to automate—and later to find patterns in—human behavior; to detect the subtle rhythms of supply meeting demand, of buying and selling, greed and fear that have driven markets since the first traders swapped shells for salt.
But somewhere along the way, as tradebots increasingly came to dominate the landscape, they began to see and react to patterns generated by each other instead. Each new generation of tradebots learns from the ghosts of trades past. The original signal—human judgments about value—grows fainter with each iteration, like a photocopy of a photocopy of a photocopy, until much of what remains is artifact and noise.
Model Collapse
The quants call this “model collapse.” For example, when trained on AI-generated text instead of human writing, chatbots like ChatGPT begin producing stale, artificial responses that grow more formulaic and less nuanced over time:
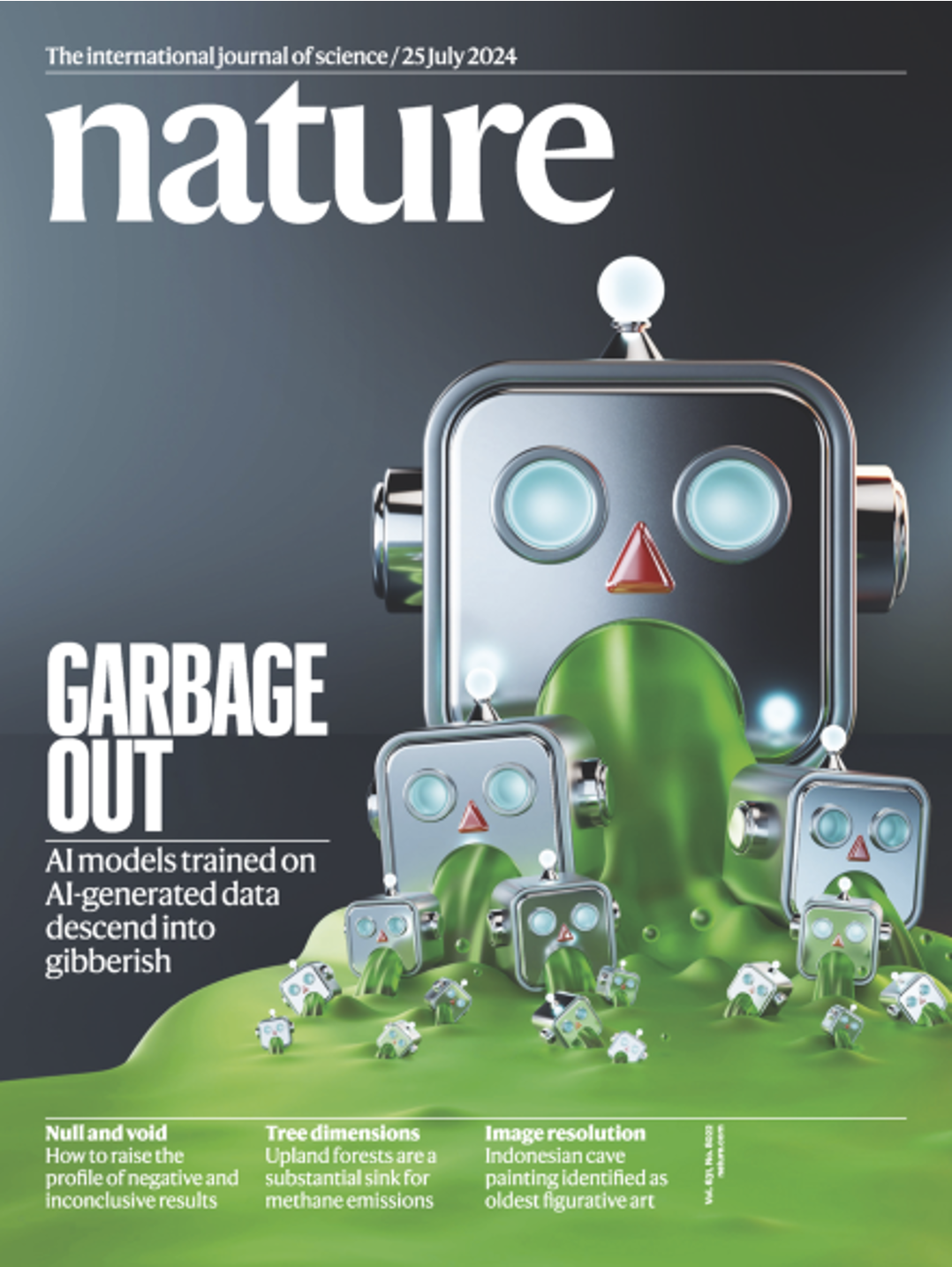
Training on AI-generated content rather than high-quality, diverse human data leads to a loss of richness, subtlety, and overall clarity in its outputs:
Model collapse is a degenerative process affecting generations of learned generative models, in which the data they generate end up polluting the training set of the next generation. Being trained on polluted data, they then mis-perceive reality…In early model collapse, the model begins losing information about the tails of the distribution; in late model collapse, the model converges to a distribution that carries little resemblance to the original one.
Skynet: Engine Of Hyperreality
This technical description parallels Baudrillard’s “precession of simulacra”—in which reality dissolves into hyperreality—and his retelling of the famous Borges fable of the map that replaces its territory. Just as Baudrillard described the progression from faithful copies to distorted copies to copies with no original, AI model collapse traces the same descent into hyperreality. Early model collapse—where the extremes of the distribution begin to fade—mirrors the initial drift from reality. Late model collapse—where the model produces a self-referential simulation bearing little resemblance to the original—is precisely Baudrillard’s hyperreal.
One study suggests that even a tiny amount of low-quality synthetic data—as low as 1%—can “poison” the training process and create a fundamental limit on how good the model can get, regardless of how much real data is added. This suggests that if synthetic data makes it into training datasets, simply scaling up real data won't solve the resulting problems—one would need to nearly completely eliminate the synthetic data to restore the model's ability to improve with scale. As AI-generated content proliferates online, future AI models will inevitably encounter increasing amounts of synthetic information, likely creating a cascade of increasingly artificial “reality”. [1]
While model collapse may (or may not) afflict individual AI models such as ChatGPT, a more profound problem emerges when we consider how our tradebots—whether of the machine learning or the more mundane pre-programmed variety—collectively shape markets. When algorithms dominate—or possibly even play a more minor role in—markets, the entire price system risks a form of model collapse.
From chatbots to tradebots, the stakes of model collapse escalate dramatically. When a given language or image generative AI model is infected by model collapse, it degrades the ability to generate coherent text or images—we lose clarity of textual or visual meaning, and usually no real harm is done.
But when the price system suffers model collapse, we lose economic meaning: we corrupt the central nervous system of human civilization. The price system coordinates society and global economic activity: the relative price structure at any snapshot in time is a compressed message transmitting vital information—about localized human wants, needs, and knowledge, technological constraints, and resource availability—across the planet and across time.
As HyperPrices detached from human economic reality proliferate and propagate recursively, both humans and tradebots respond to these synthetic price signals, creating a cascade of increasing abstraction—hyperreality. Each iteration moves us further from the “before time”—when economic signals emerged from genuine human exchange. The greater the algorithmic involvement in markets, the more these essential signals become corrupted or lost—until our markets risk becoming a self-referential hall of mirrors, where algorithms chase algorithmic shadows, and each price reflection grows more distorted than the last.
Conclusion
Our economic compass hasn’t just lost its true north—we’re increasingly living in the map rather than the territory. Our algorithms are generating a Financial Matrix dangerously disconnected from human activity and the physical world of factories and farms.
Up Next
[1] Yes, we are aware of counterarguments such as Is Model Collapse Inevitable? Breaking the Curse of Recursion by Accumulating Real and Synthetic Data. This doesn’t fundamentally change our point about price formation.
Great article. This essay outlines some possible outcomes our current phony economy might produce:
https://swiftenterprises.substack.com/p/the-great-decentralization