Black Box Bedlam
The Rise of SkyNet: The Algorithmization Of Finance Part IV
Skynet goes on-line August 4th, 1997. Human decisions are removed. Skynet begins to learn at a geometric rate. Skynet becomes self-aware at 2:14 a.m. Eastern time, August 29th. In a panic, they try to pull the plug.
New to the Sorcerer’s Apprentice series? For essential context, start with our foundational posts on Hyperreality and the Financial Matrix, or explore the full Table Of Contents.
Originally released privately on January 13, 2021. Updated, revised, and expanded for public release on October 21, 2024.
Ten Milestones On The Road To Hyperreality: Contents
1. The Metamorphosis Of Money
2. Keynes' Kaleidoscope
3. Central Banks’ Money Printer Go BRRRR
4. Silicon Shadows
5. The Rise of Skynet <== YOU ARE HERE
6. The Institutionalization Of Finance
7. The Symbolic Alchemy Of Risk
8. “We’re All Quants Now”
9. Social Media & “The Ecstasy Of Communication”
10. The Consumerification of Finance
Imagine a world where AI weaves the fabric of commerce, spinning prices from the threads of data like a cosmic loom, rendering human concepts of “expensive” and “cheap” as archaic as carrier pigeons in an age of satellite communication. Here, prices exist as mere numerical hallucinations from the digital dreamscape of ChatGPT: abstract ethereal digits untethered from reality.
Part I introduced these HyperPrices through a thought experiment set in an imaginary Costco. Part II thrust us into the unsettling reality of HyperPrices in our own world—from a $24 million Amazon book to a chaotic penny-pricing debacle. Part III revealed that Skynet is already online in the financial markets, and humans are in retreat: our semi-autonomous digital decision-makers increasingly sideline human judgment.
Now we confront some of the chaotic consequences of this algorithmic takeover. Like Mickey Mouse enchanting brooms in the Sorcerer's Apprentice, we’ve conjured forces that operate beyond our full control or comprehension—and unleashed them in the heart of our financial markets and economy. While the user interface maintains a façade of familiarity, these forces have fundamentally rewritten the economy’s operating system, giving birth to the Financial Matrix—a system in which the prices flashing across our screens represent...what, exactly?
Smart Elevators & Emergency Stop Buttons
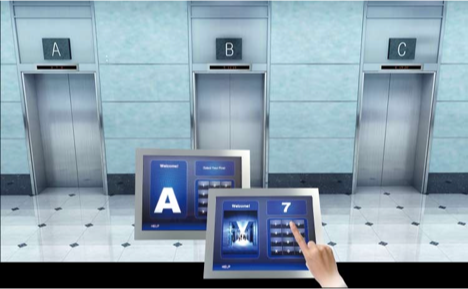
In one of his thought-provoking talks on algorithms, Kevin Slavin (formerly of MIT Media Lab) introduces the example of destination-control elevators (at approximately 11:25 minutes into the video):
So, this is the latest in elevators.
I don't know how many of you are big elevator enthusiasts or users…but you know elevators in their conventional construction are extremely inefficient, right?
Not at all how a computer would design them, right?
Because it's a bunch of people and some of them get into this one, some of them get into this one, and then these ones want to go here, and so sometimes it takes five minutes to get there, sometimes it takes one minute there, and there's an algorithm called the bin packing algorithm that says actually, if you know where everybody's going to go, you can load up these different bins differently to get people to their destination quicker.
All you have to do is have everybody announce to the computer before they get in the elevator where they're going and put them in the elevator and then just take them there, right?
And all of that's fine, except that all of a sudden you're in a metal box that unlike any other elevator you've ever been in and it has no buttons.
No buttons to press except for one.
And it says STOP.
And if the effects of algorithms are somehow abstract to you, up until now, they become more concrete when you're in a sealed metal box with nothing to press except that button.
Despite the system’s efficiency, some passengers experience anxiety and a sense of powerlessness in these elevators without familiar manual controls.
Flash Crash Of 2010: When Skynet Glitches
The unease felt in algorithmically-controlled elevators hints at a deeper fear concerning our relationship with black box technology generally: what happens when complex, semi-autonomous systems we’ve built—but don’t fully understand or control—fail catastrophically? The 2010 Flash Crash—when markets briefly entered the Twilight Zone—provided a chilling answer:
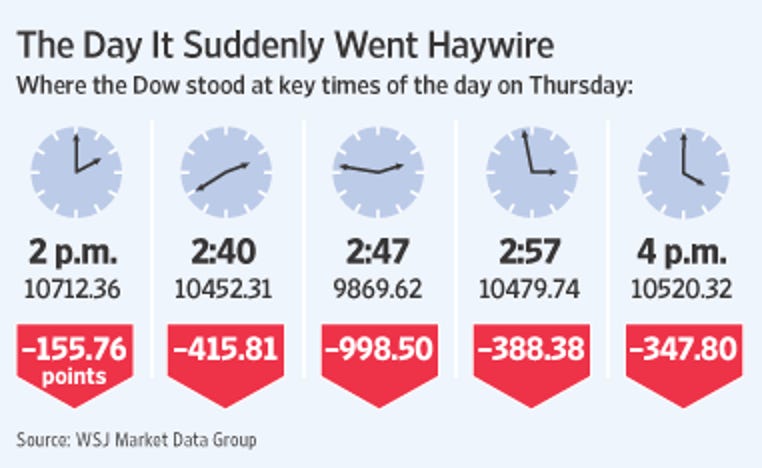
Within five minutes, $1 trillion—nearly 10% of the market’s “wealth”—was vaporized before rocketing back up a few minutes later, all without human intervention.
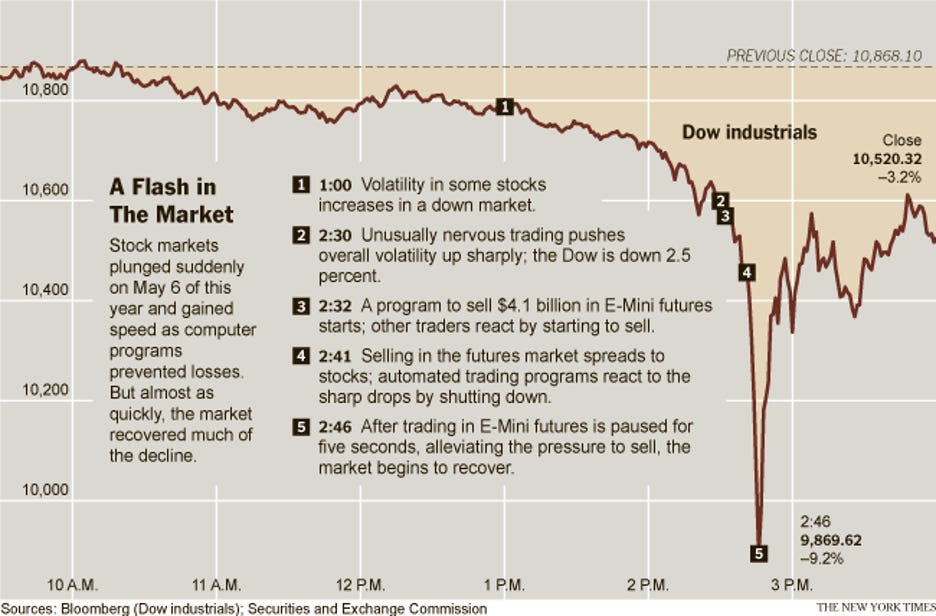
As if trapped in a runaway elevator, market participants could only watch in horror as numbers plummeted on their screens, powerless to intervene in the face of this algorithmic storm. The panic was palpable on Wall Street; the following CNBC clip captured the day's chaos:
This event has been labeled a “flash crash” and likely resulted from Skynet “gone wild”—creating a lightning-fast, high-volume feedback loop of buying and selling:
The algo-driven panic was exacerbated by an abrupt liquidity drought—at least partially due to “kill switches”, the HFT equivalent of the algorithmic elevator’s emergency “STOP” button, programmed to shut down algorithms when volatility spikes or trading volumes collapse.
The result was vertigo far more severe than in any elevator freefall: shares of real companies traded as erratically as prices of obscure fly genetics textbooks on Amazon. Blue-chip stocks like Procter & Gamble whipsawed between a penny and $100,000:
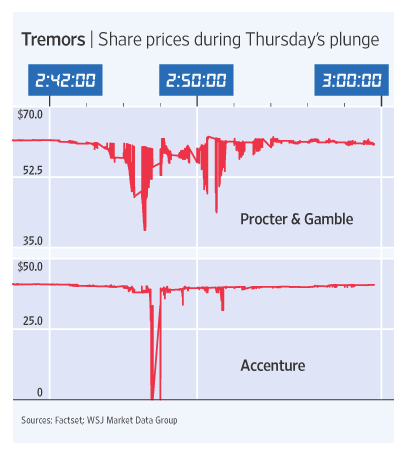
“It can't be there. That is not a real price.” Jim Cramer exclaimed exasperatedly about Proctor & Gamble—unwittingly hinting at the deeper truth of HyperPrices. “The machine obviously broke, the system obviously broke,” Cramer said, disgust evident in his voice. His colleague Erin Burnett, however, was quick to grasp the broader implications: “But do you realize the fact that after all this, that that could have just happened is an absolutely stupendous story?”
Mistakes Were Made, Lessons Were Learned
Though the most extreme trades were ultimately voided and the wild price swings quickly halted, Burnett's astute journalistic instincts cut to the heart of the matter. The prevailing reaction—exemplified by Cramer but shared by most professionals to this day—was to dismiss the incident as an isolated technical malfunction, a bug in the machine.
This widespread view—while understandable and superficially reassuring—misses the profound significance of what had transpired. The Flash Crash offered our first real glimpse into the world of HyperPrices, where algorithmic interactions in the Financial Matrix are able to detach asset prices from any anchor to the real world.
Perhaps most alarmingly, to this day no one fully understands what really transpired during the 2010 Flash Crash—what algorithms were at play, what triggered them, or how they interacted with one another. These algos are called “black boxes” for good reason—we’ve written abstract rules that we are incapable of reading. As Slavin observed:
And the really jarring thing in all of this on a sort of grand theoretical level is that nobody even could say why, because all we had left at this point is just sort of, you know, monitors with numbers moving up and down and a big red button [as in the elevator] that said STOP.
A decade later, the Flash Crash has largely faded from public consciousness, relegated to a curious footnote in financial history. Yet the incident's implications—as Burnett noted—remain profound and largely unaddressed. Her incisive question continues to echo, unanswered and unheeded.
A Warning Unheeded
In response to such concerns, one might argue: “Surely we've learned our lessons. Technology has advanced exponentially—we even have AI now. We’ve implemented a suite of measures such as Limit Up-Limit Down circuit breakers precisely to prevent such incidents, and we haven't suffered any major flash crashes since 2010—not even during the intense COVID sell-off in March 2020.”
Unfortunately, that is not true in general, and it certainly doesn’t describe what took place during COVID, as we will soon see. The types of algorithms that sparked that “stupendous” moment in 2010 haven't disappeared—they've proliferated, evolving into even more complex and opaque systems that now dominate our markets. So no, we have most assuredly not learned our lesson—or at least, not the correct one.
This is evidenced by a host of less dramatic flash crashes that have occurred since 2010 across most asset classes that employ algorithms—from treasuries to FX to commodities to cryptocurrencies. We've compiled a select chronology of the most significant incidents (found here), but suffice it to say that Skynet’s extreme HyperPrices are far from “black swan” occurrences. In fact, one famous study of the “ultrafast machine ecology” found 18,000 “mini flash crashes” and “mini flash spikes” between 2006 and 2011. Even as of 2017, these “mini flash crashes” occurred in single name stocks over 12 times per day, while FX markets suffered flash crashes bi-weekly.
More recently—in February and December 2018—several notable flash crashes further exposed the ongoing fragilities inherent in entrusting our investment decisions to Skynet. Cramer again:
I mean, this market, I don't want to call it broken. Because that sounds scary. But we're having, like, mini flash crashes every, like, 15 minutes.
Jefferies felt compelled to pen a technical primer titled “When the Market Moves The Market”—an explanation of the new computerized market structure that self-referentially precipitated these hyperreal 2018 mini-crashes. In 2018, as in the 2010 Flash Crash, market movements—likely triggered by algorithmic trading—prompted cascading automated responses from other algorithms, creating a self-reinforcing virtual feedback loop detached from reality. In other words, HyperPrices—echoing our imaginary Costco and our very real Amazon marketplace scenarios.
COVID: Algos Gone Wild
“But algos functioned perfectly during COVID!” Again, no. Algos throughout our economy generally experienced glitches as a result of the COVID “black swan”, as the MIT Technology Review observed:
But [COVID] also affected artificial intelligence, causing hiccups for the algorithms that run behind the scenes in inventory management, fraud detection, marketing, and more. Machine-learning models trained on normal human behavior are now finding that normal has changed, and some are no longer working as they should…Some say “automation is in tailspin…” What’s clear is that the pandemic has revealed how intertwined our lives are with AI, exposing a delicate codependence in which changes to our behavior change how AI works, and changes to how AI works change our behavior.
COVID: Market Dysfunction
“OK, real world algos misfired, but the algos in the financial markets are the most sophisticated and lucrative of them all, and they worked fine. Markets sold off—which one would expect given the circumstances—but at least the algorithms and market structure were fine, right?” Wrong.
While the COVID selloff—the fastest plunge from a record high into bear market territory ever—was triggered by a tangible real-world event (the pandemic) rather than a self-referential hyperreal one (like the self-perpetuating algo-algo interactions in the Amazon book pricing loop or the December 2018 flash crash), and though it didn't devolve into a full-blown algorithmic flash crash, the entire market structure did indeed begin to unravel.
Even US Government Treasuries themselves—the alleged “risk free” asset underpinning all other assets—exhibited extreme trading anomalies, including a blow-out in spreads between “on the run” and “off the run” Treasuries. As Bank of America helpfully pointed out: “The US Treasury market is the bedrock for all other financial markets. It is the world’s risk-free rate and allows the US government to fund itself. If the US Treasury market experiences large-scale illiquidity it will be difficult for other markets to price effectively and could lead to large-scale position liquidations elsewhere.”
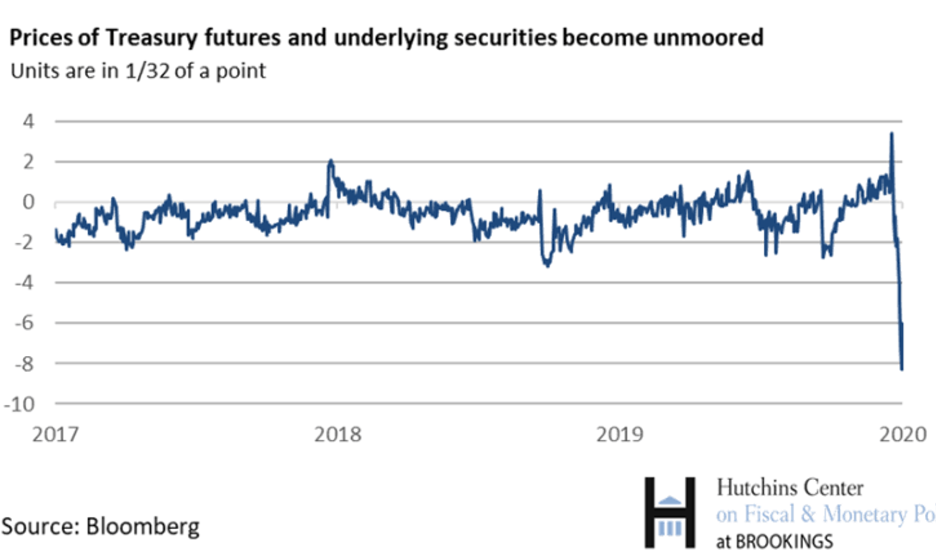
Money Printer Go BRRRR
As a result of the COVID-related glitches in the Matrix, the Fed promptly “took aggressive, unprecedented actions to restore market liquidity and preserve the special (“risk free”) role of Treasury securities.” In fewer words: Money Printer Go BRRR.
This emergency bailout amounted to $4 trillion—roughly double the 2008 response—and also included “by any means necessary” policies such as expanding QE to include corporate debt, flooding the market with unprecedented repo liquidity backstops, backstopping money market mutual funds in response to “bank runs”, and so on—to prevent a complete financial system meltdown. Markets not only rapidly returned to “normal” as a result of this drug injection, but entered a frenzied state of euphoria:
This drug-induced mania culminated in a manic blowoff—Mr. Market’s Schizophrenic Break—later in the year.
It strains credulity to suggest that any facet of our financial market plumbing—but particularly Skynet—passed the COVID test with flying colors. Just as helicopter parents anxiously hover over their progeny—ready to swoop in at the merest hint of adversity—our financial markets are subjected to a different breed of airborne oversight: one where the Federal Reserve—channeling the spirit of “Helicopter” Ben Bernanke—perpetually hovers, primed to unleash a deluge of liquidity at the slightest market hiccup.
This ceaseless Fed vigilance renders it nearly impossible to gauge the true resilience of our markets and their algorithmic underpinnings. The COVID crisis—far from serving as a genuine stress test—merely showcased another instance of the Fed firing up its monetary choppers at the first sign of turbulence. Like overprotected children who never develop the fortitude to weather life's storms, Skynet—and by extension, the markets it governs—remains untested and alarmingly fragile.
Black Swans? Or White Swans?
And yet despite virtually unlimited liquidity support and never-ending “emergency” bailouts post-2008, we’ve still experienced a litany of these algo-driven extreme HyperPrice events. These incidents occur with sufficient frequency that we can no longer dismiss all of them as mere machine malfunctions, as Cramer did a decade ago. And they persist even as the Fed attempts to eliminate price “discontinuities” and “air-pockets” and ensure “liquid and continuous markets” for Skynet—conditions critical for quant/algo models to function properly.
The Fed Is Training Skynet As Skynet Trains The Fed
Our “machine learning” algorithms are being trained to rely on bailouts raining down from the sky and to expect swift reversals of selling bouts and illiquidity. This conditioning emboldens the animal spirits—or perhaps more accurately, the algorithmic spirits—leaving them woefully unprepared for real-world stresses and sowing the seeds of greater systemic fragility.
The Fed, held hostage by Skynet, has also become conditioned like Pavlov’s dog to react to Skynet’s whims—rushing to prevent or intervene during temper (or taper) tantrums. We are cultivating a financial ecosystem as brittle and hyperreal as it is dependent on the symbiosis between the Fed and Skynet.
Skynet Terraforming The Price System
The persistence and prevalence of these “black swans” not only in the financial markets but across most sectors of the economy employing algorithmic pricing—despite safeguards specifically designed to prevent them—points to a disturbing reality: while software bugs and inadequate quality control undoubtedly play a role at times, the core issue runs far deeper.
Skynet’s influence—though most acutely visible during flash crashes and flash meltups—is not limited to these extreme events. These algorithmic interactions are continuously terraforming the price system, generating self-referential HyperPrices divorced from reality—even when the impact is not immediately obvious. Flash crashes and flash spikes are merely visible ripples atop the surface of this pervasive phenomenon. Beneath the surface, Skynet functions as an engine of hyperreality at the heart of financial markets and the economy.